
On the other hand, with an OTP verification system, virtual assistants can ensure that only verified users schedule appointments in your facility. To enhance healthcare services, it is very imperative to acquire patient feedback. Deploying a chatbot for healthcare is beneficial to understand what your patients think regarding your hospital, treatment, doctors, and overall experience of them via simple automated conversation.
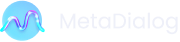
Chatbots can help physicians, patients, and nurses with better organization of a patient’s pathway to a healthy life. Nothing can replace a real doctor’s consultation, but virtual assistants can help with medication management and scheduling appointments. AI chatbots with natural language processing (NLP) and machine learning enabled help boost your support agents’ productivity and efficiency using human language analysis. You can train your bots to understand the language specific to your industry and the different ways people can ask questions. So, if you’re selling IT products, then your chatbots can learn some of the technical terms needed to effectively help your clients. As healthcare technology advances, the accuracy and relevancy of care bots as virtual assistants will also increase.
4 Collect Patient Information
Users can communicate with chatbots through text, microphones, and webcams. Making appointments is one of the activities that is done most frequently in the healthcare industry. However, due to issues like slow applications, multilevel information requirements, and other issues, many patients find it difficult to utilize an application for booking appointments. The employment of chatbots in the healthcare sector has turned out to be beneficial for the sector in many ways. In no way are these bots supposed to replace doctors or other healthcare professionals; rather, they are meant to support them and enhance their effectiveness. GDPR compliance requires an active patient’s consent before storing any of their personal information in the database.
- You also know that travelers can have anxiety about even the little things.
- Healthcare chatbots can locate nearby medical services or where to go for a certain type of care.
- Accenture estimates that by 2026, AI applications could potentially generate $150 billion in annual savings for the U.S. healthcare system.
- The process of filing insurance inquiries and claims is standardized and takes a lot of time to complete.
- Managing patient intake is facilitated by the healthcare staff; however, it has several shortcomings.
- AI Chatbots are very helpful for the healthcare sector; they reduce people’s workload.
A website might not be able to answer every question on its own, but a chatbot that is easy to use can answer more questions and provide a personal touch. Without a clear path to find solutions, patients searching for symptoms on your website may leave feeling frustrated and without the help they need. You visit the doctor, the doctor asks you questions about what you’re feeling to reach a probable diagnosis. Based on these diagnoses, they ask you to get some tests done and prescribe medicine. Chatbots are transforming the healthcare sector with their several use cases. This is why healthcare has always been open to embracing innovations that aid professionals in providing equal and sufficient care to everyone.
Driving more sales with WhatsApp chatbots
Just because a bot is a..well bot, doesn’t mean it has to sound like one and adopt a one-for-all approach for every visitor. An FAQ AI bot in healthcare can recognize returning patients, engage first-time visitors, and provide a personalized touch to visitors regardless of the type of patient or conversation. Undoubtedly, the accuracy of these chatbots will increase as well but successful adoption of healthcare chatbots will require a lot more than that. It will require a fine balance between human empathy and machine intelligence to develop chatbot solutions that can address healthcare challenges. Leveraging chatbot for healthcare help to know what your patients think about your hospital, doctors, treatment, and overall experience through a simple, automated conversation flow.
- These frequently asked questions are one of the most prevalent aspects of any website.
- Patients may sustain serious injuries or even pass away if the AI chatbot is unable to comprehend the exact situation.
- Chatbots’ key goal is to provide immediate assistance when clinicians aren’t available, so adding targeted information that can be delivered upon request will make an assistant more helpful.
- Instead, having a chatbot makes the job of extracting records quick and easy all while staying secure.
- A chatbot is able to walk the patient through post-op procedures, inform him about what to expect, and apprise him when to make contact for medical help.
- They can also be programmed to answer specific questions about a certain condition, such as what to do during a medical crisis or what to expect during a medical procedure.
One of the use cases of chatbots for customer service is offering self-service and answering frequently asked questions. This can save you customer support costs and improve the speed of response to boost user experience. By engaging with patients regularly, chatbots can help improve overall health outcomes by promoting healthy behaviors and encouraging self-care. Chatbots can help bridge the communication gap between patients and providers by providing timely answers to questions and concerns. 24/7 access to care, which is especially beneficial for those who live in rural areas or have limited transportation options.
Ready to Build Your Chatbot?
Utilizing artificial intelligence and natural language processing, these digital assistants provide patients with the ability to receive medical advice from the comfort of their own homes. The problem with chatbots in healthcare is that doing simple activities and answering basic queries no longer delivers a satisfying user experience. Ideally, healthcare chatbot development should focus on collecting and interpreting critical data, as well as providing tailored suggestions and insights. Medication assistance is a prime example of how Generative AI can revolutionize the healthcare industry. By analyzing customer data, Generative AI chatbots can send timely reminders to patients, ensuring they never miss a prescription refill.
Artificial intelligence and the future of health care – UCLA Health Connect
Artificial intelligence and the future of health care.
Posted: Thu, 25 May 2023 07:00:00 GMT [source]
A US-based care solutions provider got a patient mobile app integrated with a medical chatbot. The chatbot offered informational support, appointment scheduling, patient information collection, and assisted in the prescription refilling/renewal. The potential of AI in healthcare is enormous, and with the right investment in research and development, it is likely to transform the industry in the coming years. As AI continues to evolve, it will be essential for healthcare providers and AI development companies to work together to ensure that the technology is used responsibly and ethically.
powerful ways how WhatsApp chatbots are helping in healthcare!
It saves you the hassle of manually adding data and keeping physical copies that you fetch whenever there’s a returning patient. REVE Chat is an omnichannel customer communication platform that offers AI-powered chatbot, live chat, video chat, co-browsing, etc. Customers expect personalized experiences at each stage of the journey with a brand. The data can be saved further making patient admission, symptom tracking, doctor-patient contact, and medical record-keeping easier. The chatbot offers website visitors several options with clear guidelines on preparing for tests such as non-fasting and fasting health checkups, how to prepare for them, what to expect with results, and more. Chatbots are integrated into the medical facility database to extract information about suitable physicians, available slots, clinics, and pharmacies working days.

A chatbot can also help patients to shortlist relevant doctors/physicians and schedule an appointment. ChatGPT, developed by OpenAI, is a large language model that can understand and respond to human language in a natural way. In customer service, ChatGPT can provide quick and accurate responses to customer’s metadialog.com questions and concerns, in a conversational and natural way. In content creation, ChatGPT can be used to generate high-quality, human-like text quickly and with accuracy. In e-commerce, ChatGPT can enhance the online shopping experience by providing personalized and efficient service to customers.
They Win Patient Trust
Chatbot has become an essential functionality for telehealth app development and is utilized for remote prescriptions and renewal. To refill the prescription, a patient types a quick request into the chat window. Thus, it helps to reduce the patient mortality rates significantly across the multiple regions where the healthcare system is integrated. We leverage Azure Cosmos DB to implement a multi-model, globally distributed, elastic NoSQL database on the cloud. Our team used Cosmos DB in a connected car solution for one of the world’s technology leaders. ScienceSoft has used PostgreSQL in an IoT fleet management solution that supports 2,000+ customers with 26,500+ IoT devices.
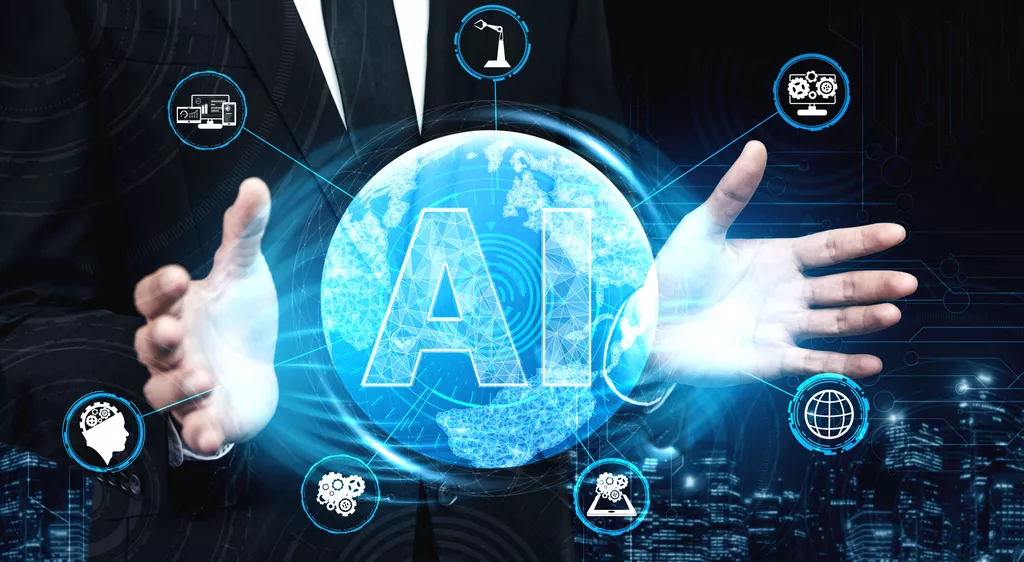
Chatbots can check account details, as well as see full reports about the user’s account. Your business can reach a wider audience, segment your visitors, and persuade consumers to shop with you through suggested products and sales advertisements. Chatbots can also track interests to provide proper notification based on the individual.
NYU Langone Health LLM can predict hospital readmissions
Healthcare is the most important industry as here the patients require quick access to medical facilities and medical information. For this, AI is used in the healthcare department as this technology has the capability to offer quick and easy support to the patients in a way that they get all the necessary information within no time. AI and healthcare integration have cut down on human labor to analyze, access, and offer healthcare professionals a list of possible patient diagnoses in a few seconds. AI-based chatbots in healthcare are created with the help of natural language processing (NLP) and this helps the chatbots to process the patient’s inputs quickly and generate a response in real-time. The main job of healthcare chatbots is to ask simple questions, for instance, has a patient been experiencing symptoms such as cold, fever, and body ache? From this, the chatbot technology analyzes the inputs of the users and offers solutions through a text or voice message.

What is the importance of AI technology in healthcare?
The emergence of artificial intelligence (AI) in healthcare has been groundbreaking, reshaping the way we diagnose, treat and monitor patients. This technology is drastically improving healthcare research and outcomes by producing more accurate diagnoses and enabling more personalized treatments.
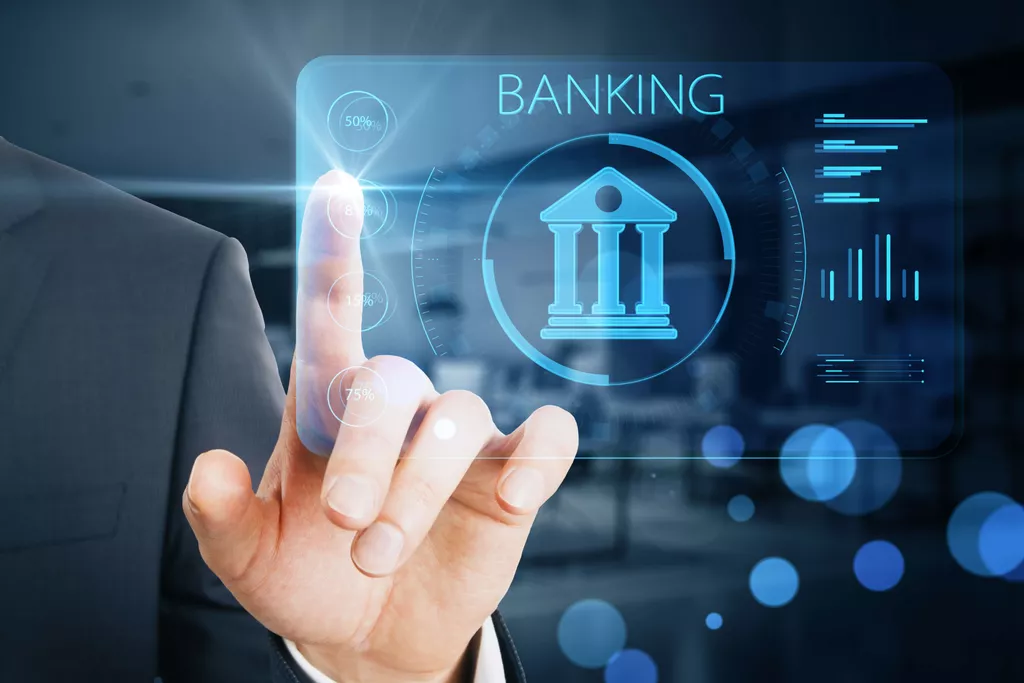
Image recognition is a subsection of computer vision, or CV, which itself is a subsection of machine learning. Within the business realm, optical character recognition is uniquely positioned to amplify daily business tasks. It can read a printed text and convert it into machine-encoded text or electronic data. For daily business processes, OCR applications can significantly reduce the time needed to manually comb through piles of documentation. Picture recognition software solutions step out as quite simple for the human brain.

For them, an image is a set of pixels, which, in turn, are described by numerical values representing their characteristics. Neural networks process these values using deep learning algorithms, comparing them with particular threshold parameters. Changing their configuration impacts network behavior and sets rules on how to identify objects. Due to their unique work principle, convolutional neural networks (CNN) yield the best results with deep learning image recognition.
What Software Does Image Recognition Software Integrate With?
In general, in the world of technology, there is always a kind of race between those who seek to exploit technological innovations illegally and those who oppose them by protecting people’s data and assets. For example, the surge of spoofing attacks leads to the improvement of anti-spoofing techniques and tools, the development of which has already become a separate specialization. The choice of the threshold is usually left to the software development customer. Lowering the similarity threshold will reduce the number of misunderstandings and delays, but will increase the likelihood of a false conclusion. The customer chooses according to priorities, specifics of the industry, and scenarios of using the automated system. It is quite easy to accurately recognize a frontal image that is evenly lit and also taken on a neutral background.
- Typically, image recognition entails building deep neural networks that analyze each image pixel.
- Most facial recognition systems work by comparing the face print to a database of known faces.
- The level of illumination and its corresponding angles could differ from place to place and depend on external factors (e.g. weather outside and movement of people within a store).
- Founded in 1998, Google is a multinational technology company that offers cloud computing, a search engine, software, hardware and other Internet-related services and products.
- In this case, the network learns on a large dataset of labeled images and distinguishes the most important patterns for different classes of images.
- In the task of image recognition, hardware and software work together to identify places, people, icons, logos, objects, buildings, and other variables that appear in digital images.
Optical character recognition (OCR) identifies printed characters or handwritten texts in images and later converts them and stores them in a text file. OCR is commonly used to scan cheques, number plates, or transcribe handwritten text to name a few. We have seen shopping complexes, movie theatres, and automotive industries commonly using barcode scanner-based machines to smoothen the experience and automate processes. Machine vision-based technologies can read the barcodes-which are unique identifiers of each item. Annotations for segmentation tasks can be performed easily and precisely by making use of V7 annotation tools, specifically the polygon annotation tool and the auto-annotate tool. A label once assigned is remembered by the software in the subsequent frames.
What are the most popular Image Recognition Software?
Such a “hierarchy of increasing complexity and abstraction” is known as feature hierarchy. To build an ML model that can, for instance, predict customer churn, data scientists must specify what input features (problem properties) the model will consider in predicting a result. That may be a customer’s education, income, lifecycle stage, product features, or modules used, number of interactions with customer support and their outcomes. The process of constructing features using domain knowledge is called feature engineering.

Much like a human making out an image at a distance, a CNN first discerns hard edges and simple shapes, then fills in information as it runs iterations of its predictions. A recurrent neural network (RNN) is used in a similar way for video applications to help computers understand how pictures in a series of frames are related to one another. Basically, you can expect your image recognition AI to be pretty bad at first. But that’s where AI companies come into play to reduce your time spent training the algorithm. Instead, they’ll train it for you, so it’s much more prepared to complete the tasks necessary once onboarded.
Uses of Image Recognition
AMC Bridge is a vendor of choice for software development services in the areas of CAD, CAM, CAE, BIM, PDM and PLM. New Platform/Technologies AdoptionAccelerate the safe adoption and integration of new disruptive technologies into your engineering software processes and offerings. Time to MarketSpeed up time to market with our expertise across the entire engineering software spectrum. So, the more layers the network has, the greater its predictive capability. Instance segmentation – differentiating multiple objects (instances) belonging to the same class (each person in a group).

Trained neural networks help doctors find deviations, make more precise diagnoses, and increase the overall efficiency of results processing. Before the development of parallel processing and extensive computing capabilities required for training deep learning models, traditional machine learning models had set standards for image processing. Here I am going to use deep learning, more specifically convolutional neural networks that can recognise RGB images of ten different kinds of animals. Founded in 1998, Google is a multinational technology company that offers cloud computing, a search engine, software, hardware and other Internet-related services and products.
Applications of image classification
If in 2019 it was estimated at $27,3 billion, then by 2025, it will grow to $53 billion. It is driven by the high demand for wearables and smartphones, drones (consumer and military), autonomous vehicles, and the introduction of Industry 4.0 and automation in various spheres. The Sobel Operator is a tool that aggregates Gaussian blur and differentiation which are used to process images. To read an image from a specific file location, use the function, imread().
Which algorithm is best for image analysis?
1. Convolutional Neural Networks (CNNs) CNN's, also known as ConvNets, consist of multiple layers and are mainly used for image processing and object detection. Yann LeCun developed the first CNN in 1988 when it was called LeNet.
Image recognition is helping these systems become more aware, essentially enabling better decisions by providing insight to the system. It’s the hidden layers — yes, that’s multiple layers — where the “magic” of image recognition processing occurs. In multiclass image recognition, the model would assign or “recognize” several labels, along with a “confidence” score for each possible label or class.
Working of Convolutional and Pooling layers
The most notable companies using this technology include Ulta, which increased its brand engagement by 700%, and Adidas, which decreased returns by 36%. Overall, retail and E-commerce are now looking to use visual identification to make shopping experiences more personalized and efficient. Therefore, it is currently present at all stages of the customer journey, including the back office. Search by image is another popular recognition instance that eases our shopping experience.

AI companies provide products that cover a wide range of AI applications, from predictive analytics and automation to natural language processing and computer vision. With 20+ years of experience and unmatched industry expertise, AMC Bridge enables digital transformation for clients in engineering, manufacturing, and AEC industries. We do it by creating custom software solutions that eliminate data silos, connect complex applications, unlock and promote internal innovation, and democratize cutting-edge technologies. In the 1960s, the field of artificial intelligence became a fully-fledged academic discipline.
PictureThis – tree, plant, or flower variety recognition.
Chopra, Hadsell, and LeCun (2005) applied a selective technique for learning complex similarity measures. This was used to study a function that maps input patterns into target spaces; it was applied for face verification and recognition. Chen and Salman (2011) discussed a regularized Siamese deep network for the extraction of speaker-specific information from mel-frequency cepstral coefficients (MFCCs). This technique performs better than state-of-the-art techniques for speaker-specific information extraction. Cano and Cruz-Roa (2020) presented a review of one-shot recognition by the Siamese network for the classification of breast cancer in histopathological images.
How AI Image Recognition Impacts Online & Offline Marketplaces – MarTech Series
How AI Image Recognition Impacts Online & Offline Marketplaces.
Posted: Thu, 20 Apr 2023 07:00:00 GMT [source]
After finishing the training process, you can analyze the system performance on test data. Intermittent weights to neural networks were updated to increase the accuracy of the systems and get precise results for recognizing the image. Therefore, neural networks process these numerical values using the deep learning algorithm and compare them with specific parameters to get the desired output.
How to Use Data Cleansing & Data Enrichment to Improve Your CRM
Most traditional image recognition models use feature engineering, which is essentially teaching machines to detect explicit lesions specified by experts. In this way, AI is now considered more efficient and has become increasingly popular. Although convolutional neural network is the big star in deep learning when it comes to image classification, artificial neural networks have also made important contributions in this field. ANNs were created to mimic the behavior of the human brain, using interconnected nodes that communicate with each other. They have been successfully applied to image classification tasks, including well-known examples such as handwritten digit recognition. Despite artificial neural networks’ early successes, convolutional neural networks have taken over the spotlight in most image classification tasks.
- The students had to develop an image recognition platform that automatically segmented foreground and background and extracted non-overlapping objects from photos.
- In the image recognition and classification, the first step is to discretize the image into pixels.
- Improvements made in the field of AI and picture recognition for the past decades have been tremendous.
- Humans recognize images using a neural network that helps them identify objects in images that they have previously learned.
- Last but not least is the entertainment and media industry that works with thousands of images and hours of video.
- On this page you will find available tools to compare image recognition software prices, features, integrations and more for you to choose the best software.
The working of CNN architecture is entirely different from traditional architecture with a connected layer where each value works as an input to each neuron of the layer. Instead of these, CNN uses filters or kernels for generating feature maps. Depending on the input image, it is a 2D or 3D matrix whose elements metadialog.com are trainable weights. After the image is broken down into thousands of individual features, the components are labeled to train the model to recognize them. Self-supervised learning is useful when labeled data is scarce and the machine needs to learn to represent the data with less precise data.

That is why object detection can be used during matches to track players and scores on the field. The algorithms can cover the diversity of medical data, including brain tumor image segmentation, mammogram mass separation, and breast ultrasound images. Processing applications can also aid in 3D imaging in biomedical applications and pathological medical imaging. Thus, malignant or cancerous elements can be identified earlier, saving countless lives and boosting diagnostic accuracy. While the overriding objective of these is automation, AI image recognition apps metamorphose into manifold benefits on the business landscape.
Which algorithm is used for OCR?
There are two main methods for extracting features in OCR: In the first method, the algorithm for feature detection defines a character by evaluating its lines and strokes. In the second method, pattern recognition works by identifying the entire character.
It is necessary to determine the model’s usability, performance, and accuracy. As the training continues, the model learns more sophisticated features until it can accurately decipher between the image classes in the training set. Instead of looking at an entire image like we do, a computer divides it into pixels and uses the RGB values of each pixel to understand if the image contains important features. Computer vision algorithms focus on one pixel blob at a time and use a kernel or filter that contains pixel multiplication values for edge detection of objects. The computer recognizes and distinguishes the image by observing all aspects of it including colors, shadows, and line drawings. But there are many insightful research papers that do a great job in the detailed technical explanations of CNN concepts in case further learning is needed.
Part L Regulations – Are You Ready for the Changes? – ThisWeekinFM
Part L Regulations – Are You Ready for the Changes?.
Posted: Mon, 12 Jun 2023 09:11:01 GMT [source]
It helps accurately detect other vehicles, traffic lights, lanes, pedestrians, and more. The security industries use image recognition technology extensively to detect and identify faces. Smart security systems use face recognition systems to allow or deny entry to people. As the layers are interconnected, each layer depends on the results of the previous layer. Therefore, a huge dataset is essential to train a neural network so that the deep learning system leans to imitate the human reasoning process and continues to learn.
- By curating your data, you’ll ensure better performance and accuracy, and achieve more optimal, relevant, and fitting data for your image classification task.
- Image recognition is highly used to identify the quality of the final product to decrease the defects.
- YOLO [44] is another state-of-the-art real-time system built on deep learning for solving image detection problems.
- The cost of image recognition software can vary greatly depending on the type, complexity, and features of the software.
- The system learns from the image and analyzes that a particular object can only be in a specific shape.
- The classification performance was evaluated on the ISIC 2017, including melanoma, nevus, and SK dermoscopy image datasets.
How does a neural network recognize images?
Convolutional neural networks consist of several layers with small neuron collections, each of them perceiving small parts of an image. The results from all the collections in a layer partially overlap in a way to create the entire image representation.
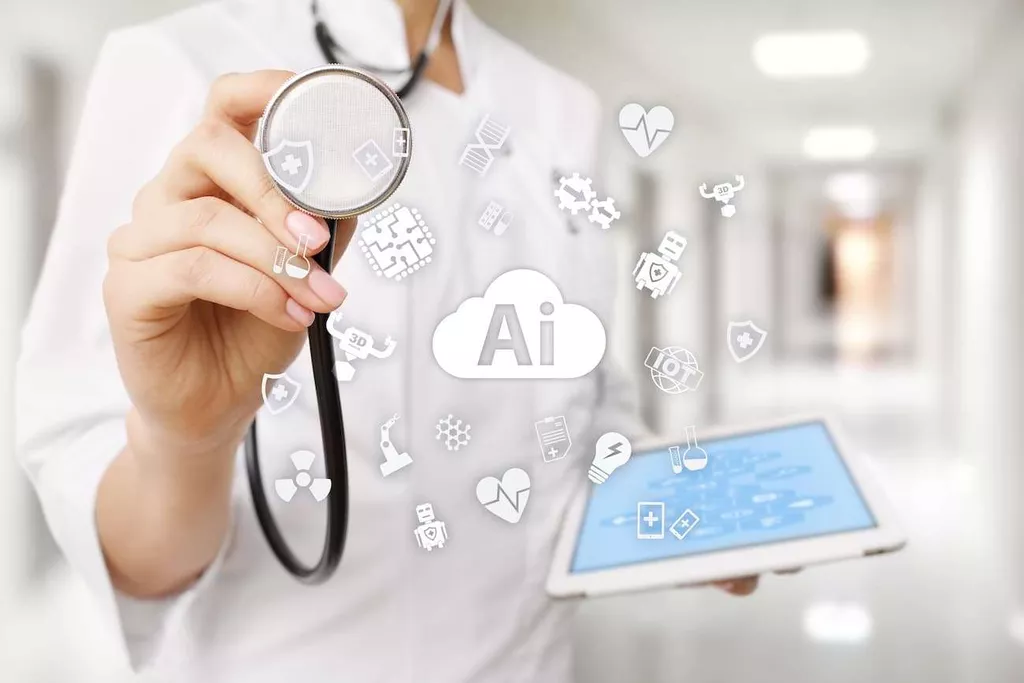
This assignment was not preceded by a theoretical part that could have, in some way, influenced the participant’s thoughts on “beauty” or any possible connotations. Participants were then asked to underline the three words (connotations) that they considered to be the most important. Nevertheless, we use the word beauty in both our everyday and specialist language, although its application to various objects or phenomena may provoke many discussions, polemics, and disputes.

For example, if we talk about the same word “Bank”, we can write the meaning ‘a financial institution’ or ‘a river bank’. In that case it would be the example of homonym because the meanings are unrelated to each other. It may be defined as the words having same spelling or same form but having different and unrelated meaning. For example, the word “Bat” is a homonymy word because bat can be an implement to hit a ball or bat is a nocturnal flying mammal also.
Application of Motion Effect Evaluation Algorithm Based on Random Forest
ArXiv is committed to these values and only works with partners that adhere to them. Our core technologies help our customers from start to finish maximize the value of their data. By helping them model their own internal data through various internal taxonomies, product codes, and proprietary internal lists, they might already have, right the way through to if they’re already using ontologies. Whether it’s large-scale analysis of biomedical literature or the enrichment of existing software infrastructures, our semantic solutions can and should play an integral part in all. Semantic Web Analytics is about delivering on these promises, empowering business users, and letting them uncover new insights from analyzing their website’s traffic.

Search engines use semantic analysis to understand better and analyze user intent as they search for information on the web. Moreover, with the ability to capture the context of user searches, the engine can provide accurate and relevant results. Cognition is emerging as a new and promising methodology in the development of cognitive-inspired computing and cognitive-inspired interactions and systems, which have the potential to have a substantial impact on our lives. The use of multimedia processing and applications to enhance human cognitive performance has great potential but requires new multimedia analysis theories to be adaptive to cognitive computational theory. It is therefore vital that new multimedia analysis applications are developed to benefit from cognitive computational theory. In the above diagram, we can see that each entity is linked to another with some attributes.
1 About Explicit Semantic Analysis
By using semantic analysis tools, concerned business stakeholders can improve decision-making and customer experience. To lower the dimensionality of a set of points, a sparse version of the random projection algorithm is utilized. In random projections, the original data is projected into a suitable lower-dimensional space in such a way that the distances between the points are roughly preserved.
- As you can see, to appear in the first positions of a Google search, it is no longer enough to rely on keywords or entry points, but to make sure that the pages of your website are understandable by Google.
- The probability-based sample, representing approximately 11.3 percent of the 2.2 million men and women in service as of October 2000, was provided by the Defense Manpower Data Center (DMDC) in California.
- Continue reading this blog to learn more about semantic analysis and how it can work with examples.
- This method is used to map sparse high-dimensional vectors to dense lower-dimensional vectors while keeping the ESA context available to other machine learning algorithms.
- They can finally bring in meetings the exact volumes they have for – let’s say – content that mentions a specific product or a category of products.
- “I ate an apple” obviously refers to the fruit, but “I got an apple” could refer to both the fruit or a product.
The meaning representation can be used to reason for verifying what is correct in the world as well as to extract the knowledge with the help of semantic representation. In this component, we combined the individual words to provide meaning in sentences. Lexical analysis is based on smaller tokens but on the contrary, the semantic analysis focuses on larger chunks. This article is part of an ongoing blog series on Natural Language Processing (NLP). I hope after reading that article you can understand the power of NLP in Artificial Intelligence.
Meaning Representation
Once that happens, a business can retain its
customers in the best manner, eventually winning an edge over its competitors. Understanding
that these in-demand methodologies will only grow in demand in the future, you
should embrace these practices sooner to get ahead of the curve. With the help of semantic analysis, machine learning tools can recognize a ticket either as a “Payment issue” or a“Shipping problem”. Therefore, in semantic analysis with machine learning, computers use Word Sense Disambiguation to determine which meaning is correct in the given context.
Previous analyses on military populations used human assisted computer analysis, but generally had less sophisticated methodologies [21]. Once the initial semantic space is created, LSA is fully automatic, permitting rapid analysis of large sets of responses. Because knowledge of word meaning is not derived from thesauri, ontologies, or hand-coding of relationships among words or among responses, bias from human coders and interpretation error is minimized.
Cognitive-Inspired Semantic Representation and Analytics for Multimedia Data
Learn how to use Explicit Semantic Analysis (ESA) as an unsupervised algorithm for feature extraction function and as a supervised algorithm for classification. The overall representation of associations related to the presence or absence of energy in feelings evoked by a beautiful object was 30 unique notions (7.673%), used in the responses for a total of 80 times (7.293%). To do that, go to your poll’s settings, open the metadialog.com “Free-form text analysis”-tab and you will be presented with two selections, Segment and Function, regarding how the analysis will be performed. For a typical employee satisfaction poll or QWL poll, the default values, “General (default) segment”, and “HR”, are the best, but it is a good idea to check all the available options. Interpretation is easy for a human but not so simple for artificial intelligence algorithms.

In Natural Language, the meaning of a word may vary as per its usage in sentences and the context of the text. Word Sense Disambiguation involves interpreting the meaning of a word based upon the context of its occurrence in a text. Hence, under Compositional Semantics Analysis, we try to understand how combinations of individual words form the meaning of the text. In the second part, the individual words will be combined to provide meaning in sentences. According to a 2020 survey by Seagate technology, around 68% of the unstructured and text data that flows into the top 1,500 global companies (surveyed) goes unattended and unused.
Leave a Reply Your email address will not be published. Required fields are marked *
This large-scale classification also requires gigantic training datasets which are usually unbalanced, that is, some classes may have significant number of training samples whereas others may be sparsely represented in the training dataset. Large-scale classification normally results in multiple target class assignments for a given test case. A typical feature extraction application of Explicit Semantic Analysis (ESA) is to identify the most relevant features of a given input and score their relevance. With nearly 1 in 5 respondents choosing to include information in the open text field, it is important to know their characteristics. Adjusted data interestingly suggest some weak patterns, albeit significant, in response to the open text field differentiated by sex, age, active-duty status, and combat occupations.
Advertising Technology (Adtech) Platform Market: Empowering … – Digital Journal
Advertising Technology (Adtech) Platform Market: Empowering ….
Posted: Fri, 02 Jun 2023 07:50:34 GMT [source]
However, investigations of potential biases in the Millennium Cohort have found a well-representative military cohort who report reliable data and who are not influenced to participate by poor health prior to enrollment [6, 10, 13–20]. Latent Semantic Analysis is a technique to transform qualitative data into quantitative information, but it has limitations, including situations where meaning is determined contextually. Additionally, it is possible that non obvious underlying relationships existed within the top-20 automatically generated clusters, which could reveal more concerns that we were unable to detect.
Nuclear energy: Twitter data mining for social listening analysis
Semantic-enhanced machine learning tools are vital natural language processing components that boost decision-making and improve the overall customer experience. The main focus of this research is to find the reasons behind the fresh cases of COVID-19 from the public’s perception for data specific to India. The analysis is done using machine learning approaches and validating the inferences with medical professionals. In the second step, an enhanced K-means clustering algorithm is used for grouping, based on the public posts from Twitter®. In the last step, latent dirichlet allocation (LDA) is applied for discovering the trigram topics relevant to the reasons behind the increase of fresh COVID-19 cases. The enhanced K-means clustering improved Dunn index value by 18.11% when compared with the traditional K-means method.
What is an example of semantic analysis?
The most important task of semantic analysis is to get the proper meaning of the sentence. For example, analyze the sentence “Ram is great.” In this sentence, the speaker is talking either about Lord Ram or about a person whose name is Ram.
What is semantic analysis in NLP?
Semantic analysis analyzes the grammatical format of sentences, including the arrangement of words, phrases, and clauses, to determine relationships between independent terms in a specific context. This is a crucial task of natural language processing (NLP) systems.
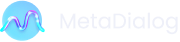
The sector in which image recognition or computer vision applications are most often used today is the production or manufacturing industry. In this sector, the human eye was, and still is, often called upon to perform certain checks, for instance for product quality. Experience has shown that the human eye is not infallible and external factors such as fatigue can have an impact on the results. These factors, combined with the ever-increasing cost of labour, have made computer vision systems readily available in this sector. To overcome these obstacles and allow machines to make better decisions, Li decided to build an improved dataset. Just three years later, Imagenet consisted of more than 3 million images, all carefully labelled and segmented into more than 5,000 categories.
- Using an image recognition algorithm makes it possible for neural networks to recognize classes of images.
- Thus, automated quality management is the result of image recognition and classification algorithms systems and applications.
- Such learning does not require specialized algorithms for each specific task.
- Once all the training data has been annotated, the deep learning model can be built.
- Meanwhile, different pixel intensities form the average of a single value and express themselves in a matrix format.
- For skin lesion dermoscopy image recognition and classification, Yu, Chen, Dou, Qin, and Heng (2017) designed a melanoma recognition approach using very deep convolutional neural networks of more than 50 layers.
Tavisca services power thousands of travel websites and enable tourists and business people all over the world to pick the right flight or hotel. By implementing Imagga’s powerful image categorization technology Tavisca was able to significantly improve the … In super-resolution imaging, the model interprets numerous low-quality images differently, leading to the treatment of all the images as having unique information. Once the variations between the photographs are analyzed, the model produces a stream of images of significantly higher quality.
The growth of computer vision
The terms image recognition, picture recognition and photo recognition are used interchangeably. More customers want to take photos of things and see where they can purchase them. After that, the filter makes a “step,” flipping by a stride length value, and multiplication of elements repeats.

So you can consider image recognition as the act of seeing, and computer vision as the understanding of what’s seen. One of the easiest entry points for any business interested in improving their operations, reducing their waste, or compiling their data into actionable insights is image recognition. Italian company Datalogic provides the IMPACT Software Suite, supporting the creation of machine vision applications. Datalogic also offers their array of sensors and machine vision cameras and hardware.
Photo, Video, and Entertainment
This extracts key points or edges from the image which can be used to identify particular objects or regions within the photo. After this step is completed then classification algorithms are applied that allow for a machine-based decision regarding what object or location has been identified within the photograph. While human beings process images and classify the objects inside images quite easily, the same is impossible for a machine unless it has been specifically trained to do so. The result of image recognition is to accurately identify and classify detected objects into various predetermined categories with the help of deep learning technology. Clarifai is a leading deep learning AI platform for computer vision, natural language processing, and automatic speech recognition.

It also uses a boosting algorithm which is meant to help have a much more accurate classification. After a massive data set of images and videos has been created, it must be analyzed and annotated with any meaningful features or characteristics. For instance, a dog image needs to be identified as a “dog.” And if there are multiple dogs in one image, they need to be labeled with tags or bounding boxes, depending on the task at hand.
What are the most mature Image Recognition Software?
The machine learning algorithm will be able to tell whether an image contains important features for that user. Image recognition is currently using both AI and classical deep learning approaches so that it can compare different images to each other or to its own repository for specific attributes such as color and scale. AI-based systems have also started to outperform computers that are trained on less detailed knowledge of a subject. For example, if you are an owner of an e-commerce business, you will benefit more from object identification and detection capabilities of the software than its facial recognition capabilities. Content moderation is another area that some businesses may need to consider carefully. Large installations or infrastructure require immense efforts in terms of inspection and maintenance, often at great heights or in other hard-to-reach places, underground or even under water.
Kidscreen » Archive » AI in action – Kidscreen
Kidscreen » Archive » AI in action.
Posted: Tue, 06 Jun 2023 15:01:48 GMT [source]
It can also be used to assess an organization’s “social media” saturation. The ability to quickly scan and identify the content of millions of images enables businesses to monitor their social metadialog.com media presence. The control over what content appears on social media channels is somewhere that businesses are exposed to potentially brand-damaging and, in some cases, illegal content.
How AI and Machine Learning Transform Banking
The following three steps form the background on which image recognition works. Evaluate 66 services based on
comprehensive, transparent and objective AIMultiple scores. For any of our scores, click the information icon to learn how it is
calculated based on objective data.

Image recognition allows computers to “see” like humans using advanced machine learning and artificial intelligence. All of these open up new growth opportunities through improving conversion rate, automating routine tasks, or upselling. Facial masks that are worn to protect from contagious viruses can reduce the accuracy of facial recognition systems. A 2020 NIST study, tested popular one-to-one matching systems and found a failure rate between five and fifty percent on masked individuals.
Current Image Recognition technology deployed for business applications
In addition, AI is already being used to identify objects on the road, including other vehicles, sharp curves, people, footpaths, and moving objects in general. But the technology must be improved, as there have been several reported incidents involving autonomous vehicle crashes. Since 90% of all medical data is based on images, computer vision is also used in medicine. Its application is wide, from using new medical diagnostic methods to analyze X-rays, mammograms, and other scans to monitoring patients for early detection of problems and surgical care. Either way, the AI face recognition software helps a lot by quickly sampling images that potentially match what is being tested. This facilitates the task of people who will assess the degree of identity of faces.
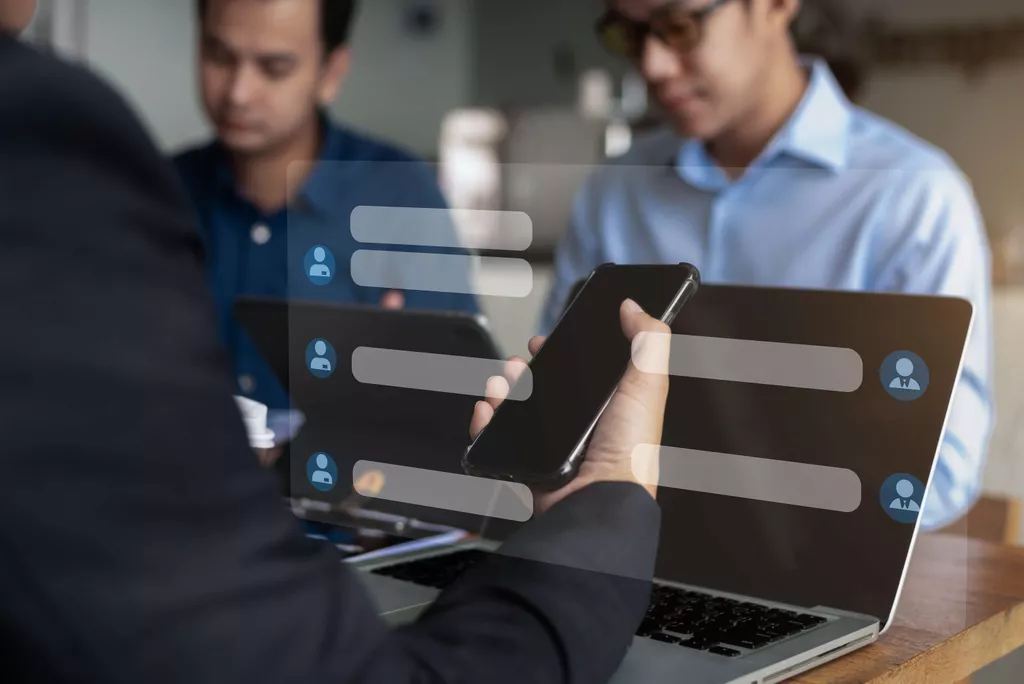
Having graduated with a History degree from the University of Birmingham, Sam has proven writing experience in biometric photography articles, marketing and events. Born in the UK, he has travelled extensively both nationally and internationally. These image reading systems have been gradually developing over the first two decades of the 21st century. OCR technology is widely used to digitize text, scan passports for automatic check-in, evaluate customer data, etc. In the near future, combined electronic chromoendoscopy with AI, the optical diagnosis will achieve optimal diagnostic accuracy that is comparable with a standard histopathologic examination. This will reduce medical costs by avoiding unnecessary resection and pathologic evaluation.
JOH Gives Clients a Data-Driven Edge Against Competitors & Increases Time to Sell
Machine learning is a subset of AI that strives to complete certain tasks by predictions based on inputs and algorithms. For example, a computer system trained with an algorithm of images of cats would eventually learn to identify pictures of cats by itself. Image recognition is a type of artificial intelligence (AI) that refers to a software‘s ability to recognize places, objects, people, actions, animals, or text from an image or video. Last but not least is the industry that has to work with thousands of images and hours of video—entertainment and media. Image recognition allows significant simplification of photo stock image cataloging, as well as automation of content moderation to prevent the publishing of prohibited content in social networks. Deep learning algorithms also help to identify fake content created using other algorithms.
AI songs flood ingsocial media – AlgoaFM News
AI songs flood ingsocial media.
Posted: Mon, 12 Jun 2023 09:47:12 GMT [source]
If you don’t know how to code, or if you are not so sure about the procedure to launch such an operation, you might consider using this type of pre-configured platform. Discover how to automate your data labeling to increase the productivity of your labeling teams! Dive into model-in-the-loop, active learning, and implement automation strategies in your own projects. Deep Learning has shown to be extremely efficient for detecting objects and classifying them. Different approaches are available and each has their own characteristics. Ambient.ai does this by integrating directly with security cameras and monitoring all the footage in real-time to detect suspicious activity and threats.
Deep Learning
Some of these uploaded images would contain racy/adult content instead of relevant vehicle images. Image recognition helps identify the unusual activities at the border areas and take automated decisions that can prevent infiltration and save the precious lives of soldiers. The primary purpose of normalization is to deduce the training time and increase the system performance. It provides the ability to configure each layer separately with minimum dependency on each other. Find out how the manufacturing sector is using AI to improve efficiency in its processes. Facial recognition is used extensively from smartphones to corporate security for the identification of unauthorized individuals accessing personal information.

Once the computer has learned these important image features and recognizes them in the training data, it can use them to classify new images that it has never seen before. Self-driving car developers use massive volumes of data from visual recognition systems, as well as machine learning and neural networks, to create systems that can drive themselves. OverFeat, Yolo, SimpleNet are some of the most common examples of detectors used in autonomous vehicles.
- While both image recognition and object recognition have numerous applications across various industries, the difference between the two lies in their scope and specificity.
- The algorithm explores these examples, learns about the visual characteristics of each category, and eventually learns how to recognize each image class.
- Once the variations between the photographs are analyzed, the model produces a stream of images of significantly higher quality.
- Image recognition is used in security systems for surveillance and monitoring purposes.
- X-ray pictures, radios, scans, all of these image materials can use image recognition to detect a single change from one point to another point.
- Image recognition is the process of identifying an object or a feature in an image or video.
What kind of algorithm is used for facial recognition?
The most common type of machine learning algorithm used for facial recognition is a deep learning Convolutional Neural Network (CNN).

A technology such as this can help to implement a customer-centered strategy. Several companies are using the sentiment analysis functionality to understand the voice of their customers, extract sentiments and emotions from text, and, in turn, derive actionable data from them. It helps capture the tone of customers when they post reviews and opinions on social media posts or company websites. Hybrid sentiment analysis systems combine natural language processing with machine learning to identify weighted sentiment phrases within their larger context. Semantic analysis is the process of understanding the meaning and interpretation of words, signs and sentence structure.
Google Cloud Announces New Foundation Models & Embeddings … – AiThority
Google Cloud Announces New Foundation Models & Embeddings ….
Posted: Tue, 16 May 2023 07:00:00 GMT [source]
In general, these feed-forward-nets consist of at least three layers (one input, one output, and at least one hidden layer) and use back-propagation as learning mechanism. The structure of the three layered back-propagation neural network is shown in Fig. TS2 SPACE provides telecommunications services by using the global satellite constellations.
The Importance Of Semantic Analysis
This avoids the necessity of having to represent all possible templates explicitly. The context-sensitive constraints on mappings to verb arguments that templates preserved are now preserved by filters on the application of the grammar rules. Rule-based technology such as Expert.ai reads all of the words in content to extract their true meaning.
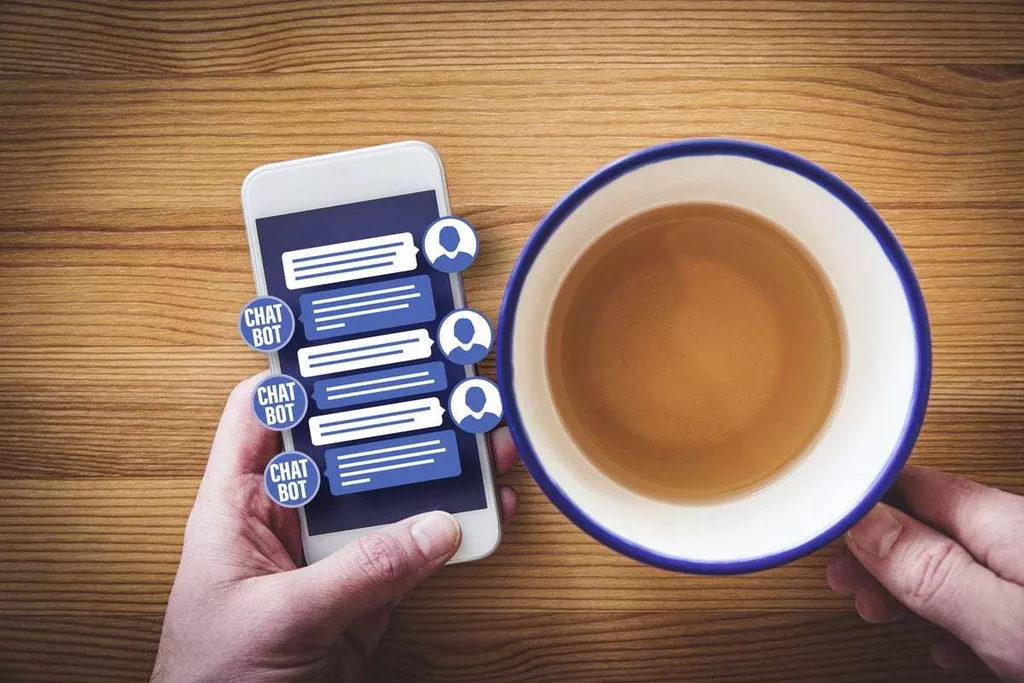
In this tutorial you will use the process of lemmatization, which normalizes a word with the context of vocabulary and morphological analysis of words in text. The lemmatization algorithm analyzes the structure of the word and its context to convert it to a normalized form. A comparison of stemming and lemmatization ultimately comes down to a trade off between speed and accuracy. In the age of social media, a single viral review can burn down an entire brand.
Contents
It is an artificial intelligence and computational linguistics-based scientific technique [11]. Semantic analysis is a term that deduces the syntactic structure of a phrase as well as the meaning of each notional word in the sentence to represent the real meaning of the sentence. Semantic analysis may convert human-understandable natural language into computer-understandable language structures. This paper studies the English semantic analysis algorithm based on the improved attention mechanism model. Semantic analysis method is a research method to reveal the meaning of words and sentences by analyzing language elements and syntactic context [12].
What are the 5 types of meaning in semantics?
Ultimately, five types of linguistic meaning are dis- cussed: conceptual, connotative, social, affective and collocative.
For example, a customer might review a product saying the battery life was too short. The sentiment analysis system will note that the negative sentiment isn’t about the product as a whole but about the battery life. In semantic analysis, machine learning is used to automatically identify and categorize the meaning of text data. This can be used to help organize and make sense of large amounts of text data. Semantic analysis can also be used to automatically generate new text data based on existing text data.
Example # 2: Hummingbird, Google’s semantic algorithm
If you’ve read my previous articles on this topic, you’ll have no trouble skipping the rest of this post. Semantic Analysis is designed to catch any errors that went unnoticed in Lexical Analysis and Parsing. Semantic Analysis is the last soldier standing before the back-end system receives the code, if the front-end goal is to reject ill-typed codes. Machine learning classifiers learn how to classify data by training with examples.
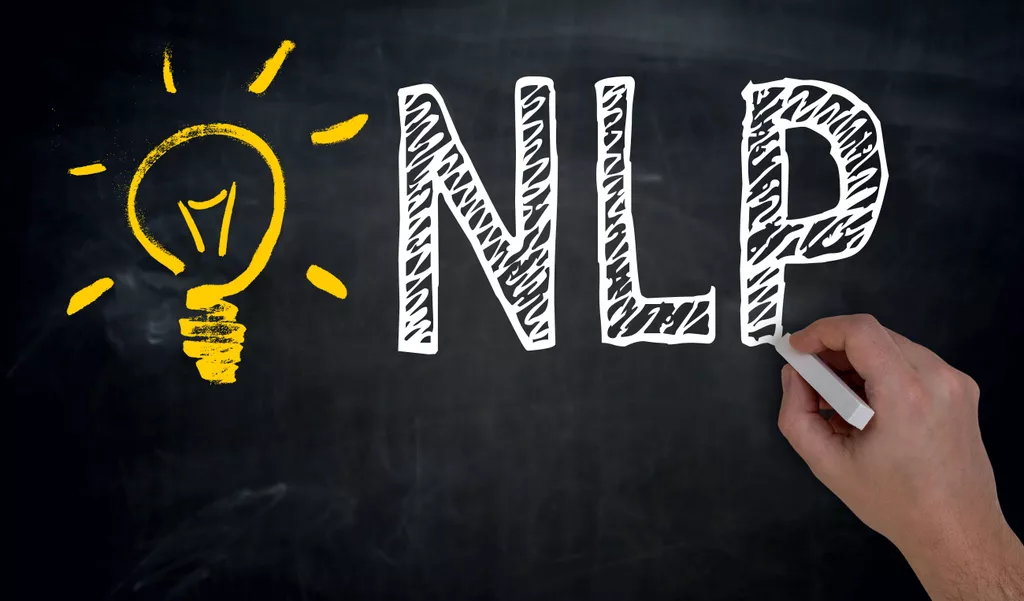
It can also determine employees’ emotional satisfaction with your company and its processes. Sentiment analysis can read beyond simple sentences and detect sarcasm, read common chat acronyms (LOL, ROFL, etc.), and correct common mistakes like misused and misspelled words. The next idea on our list is a machine learning sentiment analysis project. Like Rotten Tomatoes, IMDb is an entertainment review website where people leave their opinions on various movies and TV series.
Named Entity Extraction
Sentiment analysis of citation contexts in research/review papers is an unexplored field, primarily because of the existing myth that most research papers have a positive citation. Additionally, negative citations are hardly explicit, and the criticisms are often veiled. There is a lack of explicit sentiment expressions, and it poses a significant challenge for successful polarity identification. Let’s look at some of the most popular techniques used in natural language processing. Note how some of them are closely intertwined and only serve as subtasks for solving larger problems.
- This book aims to provide a general overview of novel approaches and empirical research findings in the area of NLP.
- You can compare this month’s results and those from the previous quarter, for instance, and find out how your brand image has changed during this time.
- That’s how Microsoft Text Analytics API analyzes a review for The Nun movie.
- The result is quick and reliable Part of Speech tagging that helps the larger text analytics system identify sentiment-bearing phrases more effectively.
- To overcome this problem, researchers devote considerable time to the integration of ontology in big data to ensure reliable interoperability between systems in order to make big data more useful, readable and exploitable.
- This is a popular way for organizations to determine and categorize opinions about a product, service or idea.
Overall, text analysis has the potential to be a valuable tool for extracting meaning from unstructured data. As technology continues to evolve, it will become an even more powerful tool for a wide range of applications. Opinion summarization is the process of extracting the main opinions or sentiments from a large number of texts. This can be done by grouping similar opinions together and identifying the most representative opinions or sentiments. C#’s semantic analysis is important because it ensures that the code being produced is semantically correct. Using semantic actions, abstract tree nodes can perform additional processing, such as semantic checking or declaring variables and variable scope.
Language translation
And from these experiences, you’ve learned to understand the strength of each adjective, receiving input and feedback along the way from teachers and peers. The letters directly above the single words show the parts of speech metadialog.com for each word (noun, verb and determiner). For example, “the thief” is a noun phrase, “robbed the apartment” is a verb phrase and when put together the two phrases form a sentence, which is marked one level higher.
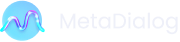
Lexical analysis is based on smaller tokens but on the contrary, the semantic analysis focuses on larger chunks. Semantic analysis employs various methods, but they all aim to comprehend the text’s meaning in a manner comparable to that of a human. This can entail figuring out the text’s primary ideas and themes and their connections. The automated process of identifying in which sense is a word used according to its context. Also, ‘smart search‘ is another functionality that one can integrate with ecommerce search tools.
Splitting the Dataset for Training and Testing the Model
Analyzing sentiments of user conversations can give you an idea about overall brand perceptions. But, to dig deeper, it is important to further classify the data with the help of Contextual Semantic Search. Intent AnalysisIntent analysis steps up the game by analyzing the user’s intention behind a message and identifying whether it relates an opinion, news, marketing, complaint, suggestion, appreciation or query. Why is, for example, the result for the NRC lexicon biased so high in sentiment compared to the Bing et al. result?
What is an example of semantic analysis?
The most important task of semantic analysis is to get the proper meaning of the sentence. For example, analyze the sentence “Ram is great.” In this sentence, the speaker is talking either about Lord Ram or about a person whose name is Ram.
What is an example of semantic process?
Semantic Narrowing
An evident example of a word that went through such a process is meat. In Old English, meat referred to any and all items of food. It could also mean something sweet, any sweet that existed at the time. As time passed, meat gradually began to refer only to animal flesh.

Few will argue that integrated AI chatbots are much better than a smart system without integration. Imagine a client of yours needs to connect with your client service area. Thanks to an integrated API, they can easily access a chatbot without needing to open a separate page. The chat may have been interrupted, and you may return to it at a later time.
How are companies using chatbot?
One of the most successful examples of using chatbots for business is providing personalized recommendations. Chatbots can analyze customer preferences and offer products or services that are tailored to them. This provides a more personal shopping experience for the customer and can increase conversions and sales.
LeadBot was designed and built to increase client engagement and optimize their lead collection process on their website and Facebook Page. Our team was responsible for conversation design, development, testing, and deployment of two chatbots on their website and Facebook Business Page. And it is understandable, given the depth of knowledge it takes for a medical professional to help a patient.
#5. Conversational AI for Messaging Apps
You can use a recruitment chatbot as a first touchpoint with your candidates. The chatbot can collect the information you need and answer candidates’ questions. The recruitment chatbot can easily cover these questions and provide detailed information about the job to the candidate.

The chatbot for education offers a wide range of chatbot use cases, making it a trustworthy, and easily accessible source of information for students. For example, suppose there’s a student who has an interest in marketing but is unsure which field really to pursue. The chatbot interacts with the student and questions them about their interests, ambitions, and current knowledge level. Based on this conversation, the chatbot for education recommends suitable courses.
Chatbot use cases in banking
You can just use a job application template or build your own recruitment chatbot from scratch. Chatbots have proven to be a fun and interactive substitute for the good-old-and-hated-online forms and CTA buttons. Offering great results organically as well as in conversational advertising.
- This is one of the biggest mistakes that companies make when deploying chatbots.
- They used our multilingual chatbot for appointment scheduling to increase their overall appointments and revenue.
- The BBC Politics chatbot allows users to get instant access to critical breaking news and frequent updates and highlights via Facebook Messenger.
- Chatbots for retail and eCommerce are evolving as they become advanced enough to tackle marketing and customer service.
- You can use chatbots to guide your customers through the marketing funnel, all the way to the purchase.
- AI isn’t new technology anymore; however, many companies are still learning how it fits into their overall business strategies.
In this section, we will cover some of those common chatbot use cases in the support process. To help you understand how chatbots are used, we’ve listed down 20 popular ways a business can use them. All the various types of intent are represented in this set of training data. These data points are used by chatbots to develop mathematical models for intent recognition.
Chatbot Use Cases for Marketing
Depending on the issue, a chatbot can serve as the first point of contact and help you route customer queries to the right departments. It’s especially useful for companies that have two or more separate teams metadialog.com that communicate with clients directly but focus on different areas of the issues. Chatbots can be good customer engagement tools, as they are always there to chat and reply quickly to user queries.
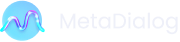
This way, the load on your staff will decrease, the quality of service will stay high, and you’ll keep customers happy. And it won’t harm the customer satisfaction your online store provides as our study on the current chatbot trends found that over 70% of buyers have a positive experience using chatbots. One of the most common support FAQs reported by customers is for refunds and exchanges.
Bots: The Digital Agents
Instead, utilize the conversation to bring the product to the customer. Furthermore, chatbots can be programmed to recall previous interactions and purchase history to provide personalized recommendations based on the buyer’s preferences. The travel industry can utilize chatbots and cater to customers 24/7. Chatbots can answer questions about tourism, generate leads, and handle customer queries. In an industry where time is of crucial importance, chatbots can prove themselves to be helpful. Hospitals and clinics won’t get overwhelmed with basic questions and queries, and on the other hand, patients will get prompt answers.
What is the use of chatbot in daily life?
- Make captions for social media posts.
- Check your homework.
- Make long articles easier to read.
- Think of business ideas.
- Write a great CV or resume.
- Ask ChatGPT to read a contract and detect areas of concern.
- Chat with ChatGPT.
- Find love with ChatGPT.
Here are the top chatbot use cases divided by category and the first to discuss is customer service. Being a customer service adherent, her goal is to show that organizations can use customer experience as a competitive advantage and win customer loyalty. The chatbot gives you suggestions for answers and even questions to ask.
Promising Lead Qualification
With the right script planning and triggers, you can create a chatbot to cross-sell and upsell your customers. Instapage asks visitors a bunch of preliminary questions before assigning them to a sales rep or proposing a plan. This is how they get such a detailed understanding of their buyer personas and efficiently convert prospects into customers. Peloton asks for the visitor’s email address right after they send their first message.
Microsoft Considers More Limits for Its New A.I. Chatbot – The New York Times
Microsoft Considers More Limits for Its New A.I. Chatbot.
Posted: Thu, 16 Feb 2023 08:00:00 GMT [source]
Employing a chatbot could truly be one of the best investments you could make to optimise your business’ conversions. Chatbots can handle online transactions and accept payments from within the bot itself rather than sending users to a different page and dragging on the payment process. Chatbots for e-commerce can identify the products & services that your customers are interested in. Keeping these in mind, the bots can recommend higher quality products that would serve the customer better. Customer service is the function to which bots have been applied the most.
eCommerce AI chatbot use case #2: Notification Bots
Just as human agents in customer service roles have specific skillsets and tasks, so too can bots be designed and built to carry out specific activities or facilitate particular journeys. In a sense, the VA acts as an orchestrator or manager of other bots who act as task workers. Ultimately a string of bots can be deployed to handle an end-to-end workflow if necessary. It’s similar in nature to a team of human agents that work across the customer journey or process to fulfill specific objectives or requirements. They can be quite general, such as a bot for FAQs or appointment setting or they can be industry-specific such as processing an insurance claim or providing bank account balances. And the customer can be an external consumer of your products and services or it can be an internal customer, like your employees.
- Another reason why companies adopt chatbot solutions is their capability to answer repetitive questions.
- With the ability to launch and align predefined conversation templates with the queries and store chat information, your team does not need to re-explain the issues.
- With REVE Chat, start a free trial of advanced customer support software and put start delivering great experiences to customers.
- You can train your bots to understand the language specific to your industry and the different ways people can ask questions.
- The feedback bot can be deployed where your customers are, be it on your website or mobile app.
- They can ask open-ended questions or share a feedback form in the chat widget.
They won’t have a way to contact their site’s visitors since the traffic is anonyms, and there isn’t a way to identify anyone. Companies usually do surveys to find out what their customers think. However, it can sometimes be hard to get people to participate in surveys. However, what happens if 1000 people are planning to come to the seminar? Bots address daily business tasks and allow the support team to focus on more complex questions. To boost your sales, one of the critical requirements you need to fulfill is customer engagement.
Is Alexa a chatbot?
Alexa Virtual Assistant – Definition & use cases
Alexa is a virtual assistant technology that employs A.I. and NLP to parse user queries and respond. It is developed by Amazon and is mostly used in Echo speakers and smartphones.